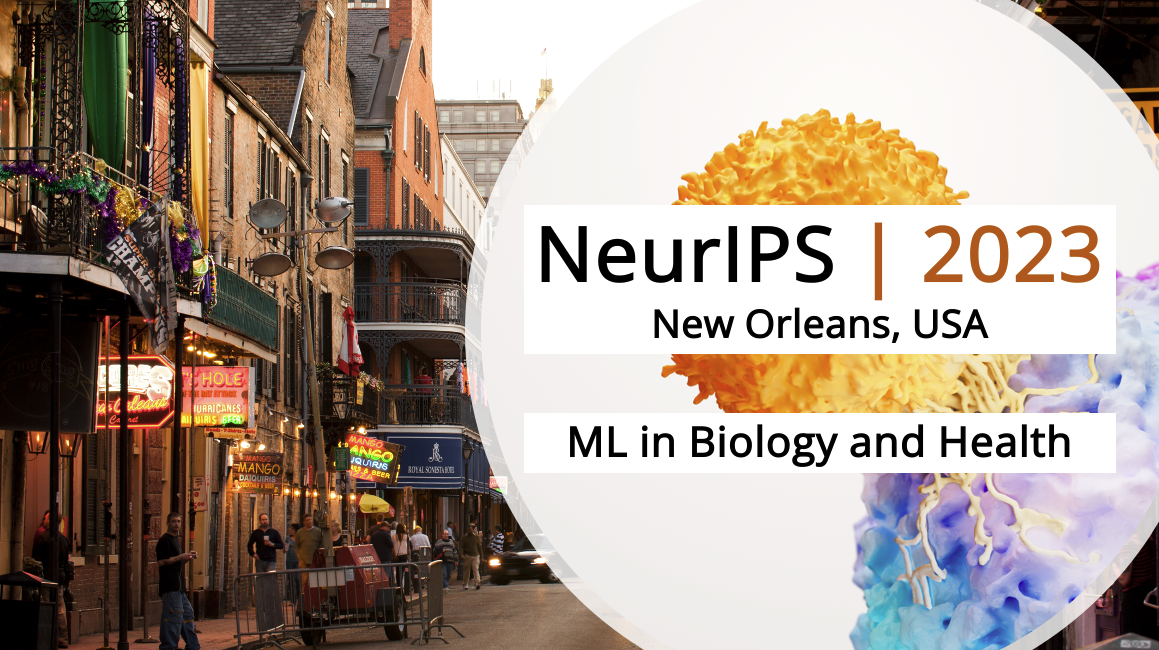
NeurIPS 2023 Health & Bio Conference Review
Unable to keep up with the deluge of amazing work happening in ML for Biology and Health at NeurIPS this year?
We’ve got you covered with a concise summary of NeurIPS 2023 content focussed at the exciting intersection of Biology, Health and AI!
Keynotes
Delusion of Scaling and Democratization of Generative Models - Björn Ommer
Björn Ommer (Stable Diff) starts us off with defining human vision as grasping things without touch and perception as a process of prediction. He argues intelligence is learning under finite resources to support research outside scaling & makes case for accessible and open models.
Systems for Foundation Models, and Foundation Models for Systems - Chris Re
In a captivating talk, Chris Ré shows us the potential of foundation models (FMs) for systems and introduces the paradigm shift behind FMs: from solve in detail, to solve in general He outlines data cleaning as a valuable example where FM have made huge strides (also OSS models)
Selected papers
De novo Drug Design using Reinforcement Learning with Multiple GPT Agents
Xinyuan Hu et al (Microsoft Research, Tsinghua University) introduce a multi GPT agent framework for de-novo drug design with RL with the goal of promoting diverse candidate generation. Agents use memory, and they show candidate inhibitors for SARS-CoV2.
Implicit Transfer Operator Learning: Multiple Time-Resolution Models for Molecular Dynamics
M Schreiner et al (Chalmers University, DTU) address the key problem of reconciling small time steps and long convergence times in molecular dynamics (MD) simulations.
Their new multi-timestep transfer operator shows self-consistent stochastic dynamics across time-scales.
xTrimoGene: An Efficient and Scalable Representation Learner for Single-Cell RNA-Seq Data
J Gong et al (BioMap & Tsinghua University) present xTrimoGene - a scalable representation learner for scRNAseq data.
Using self-supervised learning and an encoder-decoder architecture, they show respectable performance on cell type annotation, response prediction and drug combination prediction tasks.
HyenaDNA: Long-Range Genomic Sequence Modeling at Single Nucleotide Resolution
Eric Nguyen et al (Stanford) present HyenaDNA a long-range, efficient single-nucleotide model with sequence windows up to an impressive 1m base pairs that is trained on self-supervised next nucleotide prediction task. They show scale benefits performance on species classification.
Protein Design with Guided Discrete Diffusion
N Gruver et al (Prescient Design) present a discrete guided diffusion approach to antibody (Ab) design that leverages multi-objective optimisation to optimize Ab properties.
They show impressive improvements in iterative experimental rounds in binding and expression of Ab candidates.
Knowledge-based in silico models and dataset for the comparative evaluation of mammography AI for a range of breast characteristics, lesion conspicuities and doses
Elena Sizikova et al (US FDA) introduce an approach for synthetic data generation to evaluate AI mammography algorithms and address limitations of evaluating against real-world data, incl biases and small dataset sizes They use their approach to evaluate AI models for mammography.
FABind: Fast and Accurate Protein-Ligand Binding
Qizhi Pei et al (Microsoft Research & Renmin University) introduce a fast and accurate method (FABind) for protein-ligand binding prediction without pocket information. Their approach combines pocket prediction and docking and achieves SOTA quantitative results on PDBbind data.
SG×P : A Sorghum Genotype × Phenotype Prediction Dataset and Benchmark
As is the case in human health, understanding the relationship between genetic background and observed outcomes (phenotypes) is of paramount importance also in plant sciences.
Z Zhang et al (George Washington University) create a Sorghum dataset (500k+ images) for studying these relationships.
AbDiffuser: Full-Atom Generation of In-Vitro Functioning Antibodies
K Martinkus et al (Prescient Design) present AbDiffuser - a generative approach to antibody design that improves protein diffusion by integrating domain knowledge and physics-based constraints
They present exciting evidence that some of their candidates were novel HER2 binders
ProteinGym: Large-Scale Benchmarks for Protein Fitness Prediction and Design
In recognition that proteins need to stay fit too, P Notin et al (Oxford University & Harvard University) introduce ProteinGym - a benchmark for protein fitness prediction that features curated deep mutational scanning & clinical datasets, relevant baseline models and metrics.
RaLEs: a Benchmark for Radiology Language Evaluations
J Z Chaves et al (Stanford) address the challenge of evaluating natural language models for radiological findings.
They introduce a benchmark and find that advances in more general domains do not necessarily translate to Radiology and highlight opportunities for future work
Disentangled Wasserstein Autoencoder for T-Cell Receptor Engineering
Tianxiao Li et al (@Yale) present an exciting advance in engineering T-cell receptors that balances maintaining the overall structure with modifying the functional site. They use a disentangled Wasserstein autoencoder that demonstrates quality & quantity of results in experiments.
Conclusion
Overall, key observations are:
- Health and bio is coming of age in the ML community (though still mostly relegated to posters)
- Sequence learning, proteomics and design tasks are emerging as areas of especially high activity and promise for ML in bio
DISCLAIMER: The above list is a personal curation that most certainly missed many key contributions (in particular the many excellent workshop & competition contributions!) and is only intended to be a starting point for your own exploration.